Machine Learning Breakthrough Enhances Glacier Lake Depth Measurement Precision
February 24th, 2025 8:00 AM
By: Newsworthy Staff
Researchers from Sun Yat-sen University have developed a novel machine learning approach using satellite data to more accurately measure glacier lake depths, providing critical insights into ice sheet dynamics and potential sea-level rise impacts.
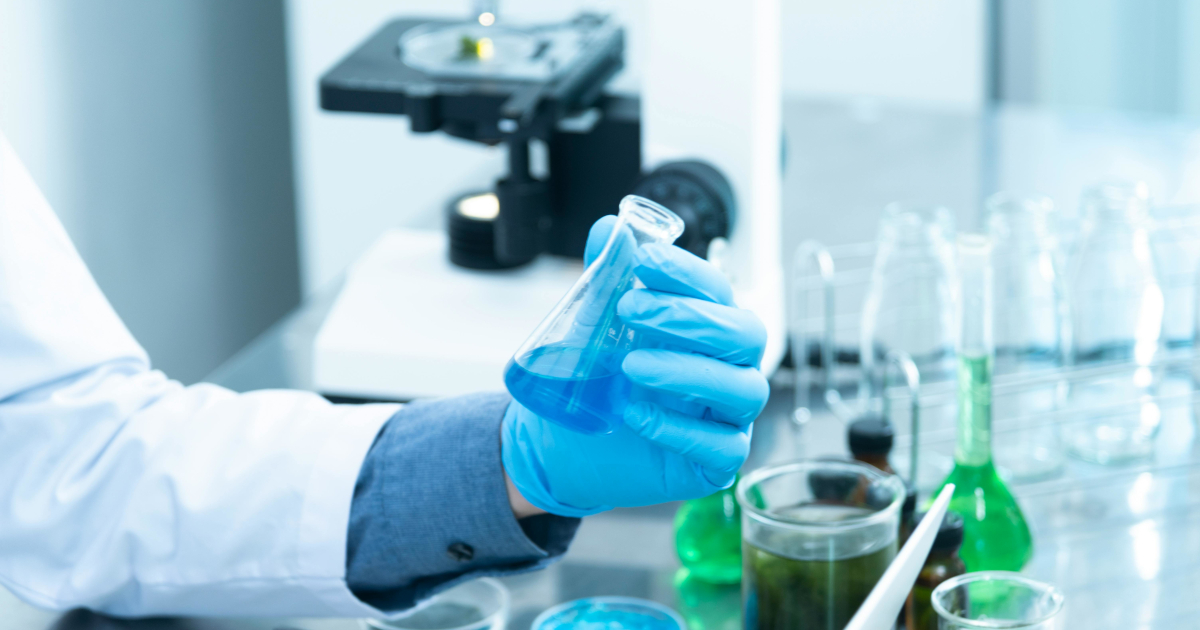
Scientists have unveiled a sophisticated machine learning method that promises to revolutionize the measurement of glacier lake depths, addressing a critical challenge in understanding global climate change. The innovative technique combines advanced algorithms with satellite imagery to provide unprecedented accuracy in mapping supraglacial lake bathymetry.
Researchers from Sun Yat-sen University developed a groundbreaking approach that integrates machine learning algorithms like XGBoost and LightGBM with data from ICESat-2 satellite and multispectral imagery. This method significantly improves depth estimation accuracy, achieving a root mean square error of just 0.54 meters when applied to Sentinel-2 satellite imagery.
The significance of this research extends far beyond technical achievement. As global warming accelerates, understanding the dynamics of supraglacial lakes becomes increasingly crucial for predicting ice sheet behavior and potential sea-level rise. Traditional measurement techniques have struggled with accuracy, particularly in deeper lakes, making this new methodology a potential game-changer for climate science.
By creating an enhanced Automated Lake Depth algorithm, the research team extracted reliable depth sample points from satellite data and developed machine learning models capable of generating highly precise measurements. The methodology was rigorously tested across seven supraglacial lakes in Greenland, demonstrating remarkable performance compared to conventional measurement techniques.
Lead researcher Dr. Qi Liang emphasized the broader implications of their work, noting that the approach offers a scalable solution for large-area monitoring. The research not only improves depth estimation accuracy but also provides a powerful tool for assessing climate change impacts in polar and glaciated regions.
An unexpected finding emerged regarding atmospheric corrections: top-of-atmosphere reflectance data performed better than atmospherically corrected data for mapping lake bathymetry. This insight suggests that current atmospheric correction methods might introduce significant measurement errors, particularly over complex surfaces like water, snow, and ice.
The research, published in the Journal of Remote Sensing, represents a significant step forward in climate monitoring technologies. By combining machine learning with advanced satellite imaging, scientists can now obtain more precise measurements of glacier lake depths, potentially refining global climate models and sea-level rise predictions.
Source Statement
This news article relied primarily on a press release disributed by 24-7 Press Release. You can read the source press release here,
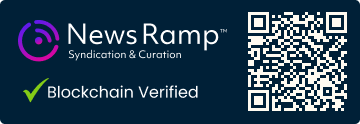